Gradient Enhanced Neural Networks for Optimization of Mars Low Reynold Number Airfoil
The project uses improved gradient-enhanced neural network as a surrogate model instead of CFD simulation calculation to predict the aerodynamic parameters of airfoils. By comparing and testing the traditional neural network, the gradient-enhanced neural network(mSANN) and the improved gradient-enhanced neural network, the superiority of the network in terms of prediction performance and convergence speed is clarified.
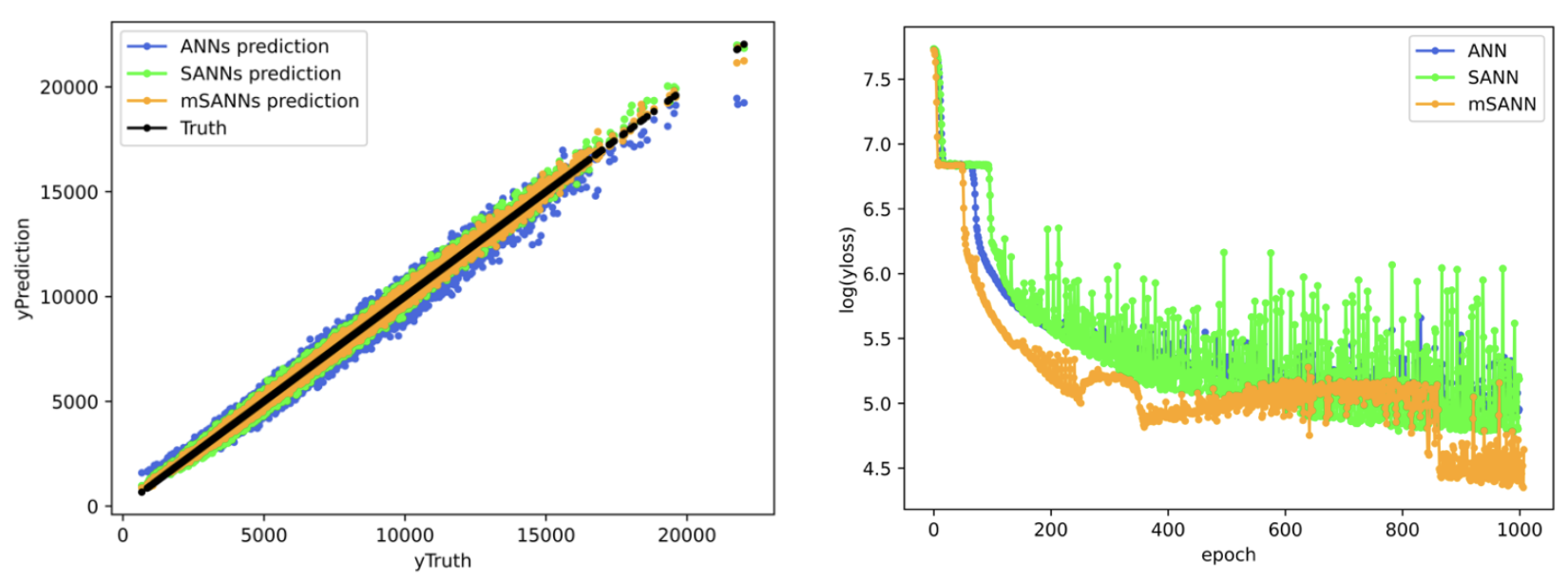
For the preprocessing of data, this paper uses singular value decomposition to parameterize airfoils and decompose airfoils into modes and modal coefficients. The inverse distance-weighted interpolation method is used to construct a constraint function to limit the modal coefficient and exclude abnormal airfoils.
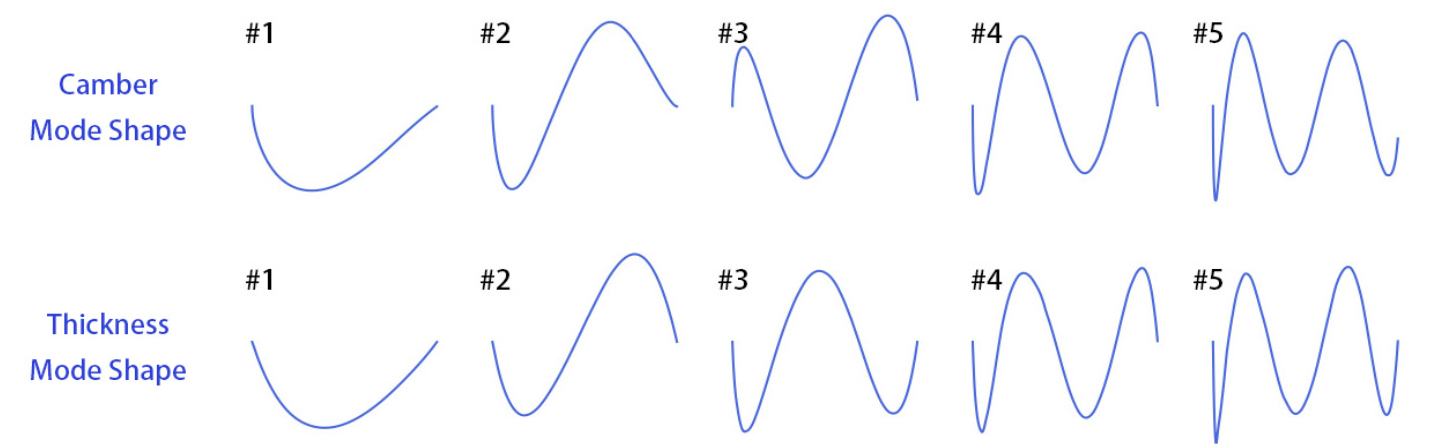
Latin hypercube sampling was used to generate airfoil samples, and ADflow was used to calculate the aerodynamic parameters and their derivatives. The results are used for the training of the improved gradient-enhanced neural network to obtain a surrogate model for the prediction of the aerodynamics, and the neural network is coupled with the optimization software package for airfoil aerodynamic optimization. Compared with the high-fidelity CFD based optimization method, the optimization results are almost indistinguishable, but the optimization time is greatly reduced.
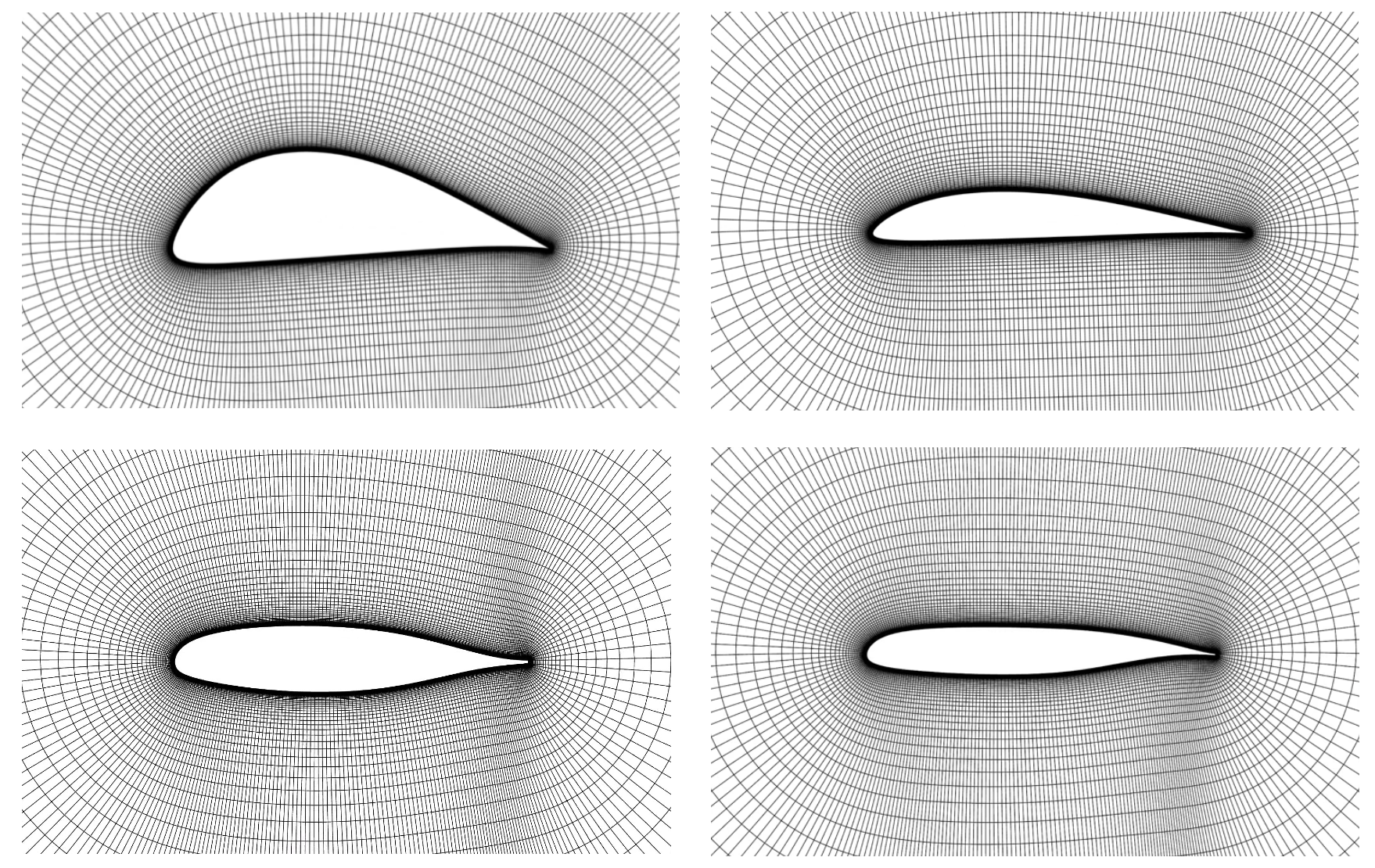